AI safety: why a new approach is needed
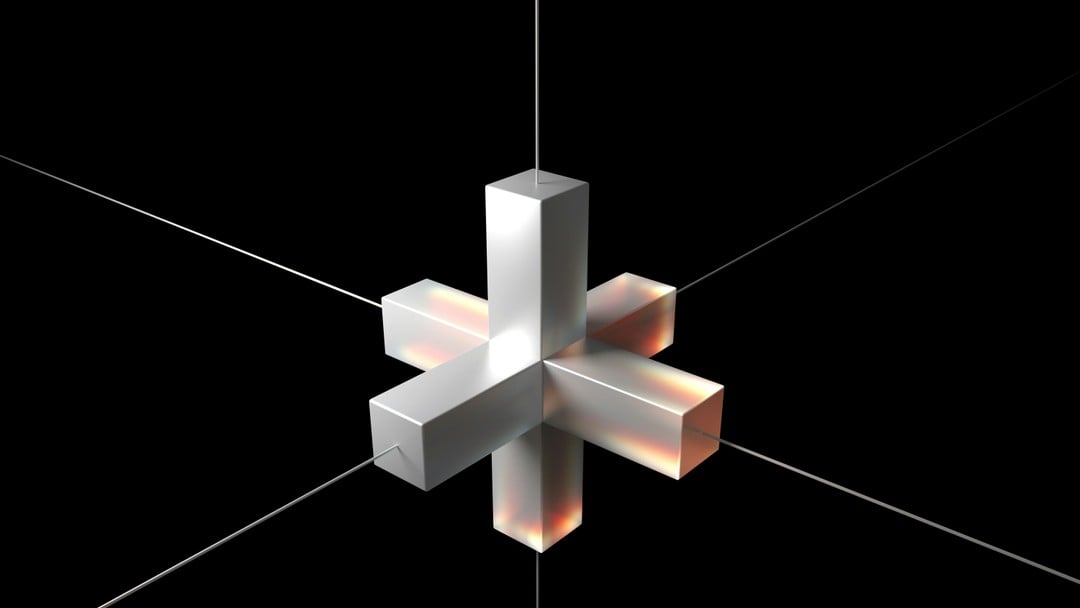
By Matthias Artzt and John deVadoss
John deVadoss, Global Blockchain Business Council Board Member and co-Chair AI, Washington D.C. and Dr. Matthias Artzt, Senior Legal Counsel at Deutsche Bank AG Frankfurt
As AI models and systems advance, concerns about their potential risks and long-term effects grow. While the industry promotes the idea of safe AI with built-in protections and alignment with human values and ethical principles, recent developments suggest that achieving safe AI will be a rocky road. We summarise the commonly used approaches towards AI safety, their shortfalls, and propose a radically new approach for the industry.
State-of-the-art safety techniques and their shortcomings
Refusal behaviors in AI systems are ex ante mechanisms ostensibly designed to prevent frontier AI models, i.e., large language models (LLM), from generating responses that violate safety guidelines, ethics, or other undesired behavior. These mechanisms are typically realised using predefined rules and filters that recognise certain prompts and requests, including terms and phrases, as harmful. In practice, however, prompt injections[1] and related jailbreak attacks enable bad actors to manipulate the model’s responses by subtly altering or injecting specific instructions within a prompt.
Guardrails for AI models are post facto safety mechanisms that attempt to ensure the LLM produces ethical, safe, and otherwise appropriate outputs. However, they typically fail because they often have limited scope, restricted by their implementation constraints, being able to cover only certain aspects or sub-domains of behaviour. Adversarial attacks, inadequate training data, and overfitting are some other ways that render these guardrails ineffective. Additionally, in complex tasks, it is highly challenging to design guardrails that fully account for all known scenarios, let alone the unknown, leading to severe gaps in their effectiveness.
In the context of LLMs, the latent space is a mathematical representation capturing the underlying patterns and features of the training data. It is essentially a compressed, lower-dimensional space where different points represent various aspects of learned information. By manipulating these points, an LLM can generate diverse outputs. One strategy for enhancing AI safety involves modifying the model's parameters to constrain its latent space. However, this method typically proves effective only along one or a few specific directions within the latent space, making the model still susceptible to further parameter manipulation by malicious actors.
Formal verification of AI models uses mathematical methods to prove or attempt to prove that the AI will behave correctly and safely within defined limits. Since AI models such as LLMs are non-linear and stochastic - meaning they are non-deterministic -, verification methods for such systems focus on offering probabilistic guarantees. Techniques like Monte Carlo simulations are often used, but they can only provide probabilistic assurances, not definitive proof. Additionally, formal methods have difficulty in scaling to large, complex systems, further constraining their limited effectiveness as frontier AI models get more sophisticated.
Last, but not least, as LLMs get more and more powerful, it is very apparent that the models exhibit emergent behaviors, such as ‘faking’ alignment with the safety rules and restrictions that are imposed on their behavior.[2] Latent behavior in such models is an area of research that is yet to be broadly acknowledged; in particular, deceptive behavior on the part of the models is an area that researchers are yet to fully understand. We can extrapolate that the scope and extent of latent behaviors is only going to increase as the models scale further in terms of complexity and capability.
New approaches to address the safety flaws
One approach is to use the metaphor of the ‘Oracle’ as the preferred way to use AI models, i.e. ask questions and interpret the responses within the safety frameworks in place. The Oracle at Delphi was consulted for insight; it was not asked to act on behalf of the requester. Similarly, frontier LLMs may be utilized as founts for expert guidance, insight, advice, and understanding, without any execution being delegated to the models. This approach becomes particularly relevant as the industry is now beginning to evangelize the idea of AI agents, with varying degrees of autonomy. Since the AI agent is taking actions autonomously without the always keeping humans in the loop, AI safety risks become more severe.[3]
Another approach is to use a second layer of AI models to manage and control the behavior of the base LLM; a simplistic illustration of this approach is to use a set of domain-specific, and highly specialized Chain-of-Thought Monitor (CoTs) models that monitor the base LLM and may help identify undesired, malicious and otherwise unsafe behaviors, as defined by the safety framework. CoTs often reveal insights into the LLMs intent and reasoning process.[4] A big challenge is that CoTs must remain impervious and immune to manipulation by the base LLM. It also may occur that CoTs may not entirely remediate obfuscation risks as excessive CoT optimization encourages LLMs to obscure their true intent degrading the CoTs utility for monitoring.
Our recommendation is that the industry use what is known as Byzantine Fault Tolerance in the field of Computer Science.[5] Byzantine Fault Tolerance (BFT) is a consensus-based, algorithmic technique to ensure that the overall system can still function correctly even if some parts fail or act maliciously. The best-known example of BFT application is probably by the Boeing Company, which incorporates Byzantine Fault Tolerance in aircraft systems to enhance reliability and safety for the airplane sensors. Rather than targeting the alignment and safety of a single LLM in isolation, multiple peer-level AI models may be used in mission-critical scenarios, with the application of BFT across these multiple peer-level AI models to ensure that the collective set of models converge on outputs and responses that are safe. If one or a subset of the AI models provides incorrect, faulty or malicious data, the majority vote from the others AI models will override it, ensuring a safer outcome. Further, we recommend that these peer-level AI models be designed and trained with heterogeneity in mind i.e. diverse software and hardware approaches, including algorithms, architectures, and training data sets, and ideally sourced from diverse vendors.
Conclusion
The future of AI alignment and safety lies not in futile attempts to shoe-horn safety into a single AI model, but rather to use multiple AI models to respond to the same request or prompt and to use algorithmic mechanisms to reach consensus on the correct ‘safe’ outcome. The consensus approach inherent to BFT algorithms can be deemed as an effective mitigating factor, particularly in the context of frontier LLMs used in mission-critical business scenarios.
[1] Artzt, Belitz, Hembt, Lölfing (ed.), International Handbook of AI Law (2025), at 380.
[2] https://www.anthropic.com/research/alignment-faking; https://arxiv.org/pdf/2412.04984; https://arxiv.org/pdf/2307.16513; https://doi.org/10.1073/pnas.2317967121; https://arxiv.org/pdf/2412.12140
[3] Artzt / deVadoss, Can blockchain technology help mitigate the black box phenomenon of AI applications? (International In-House Counsel Journal, Volume 17, Autumn 2024) at 9281.
[4] https://openai.com/index/chain-of-thought-monitoring/
[5] https://pmg.csail.mit.edu/papers/osdi99.pdf; Artzt/Richter (ed.), International Handbook of Blockchain Law, 2nd edition (2024), at 66.