Synthetic data generation: study, led by Professor Ana Beduschi from the University of Exeter, calls for guidelines
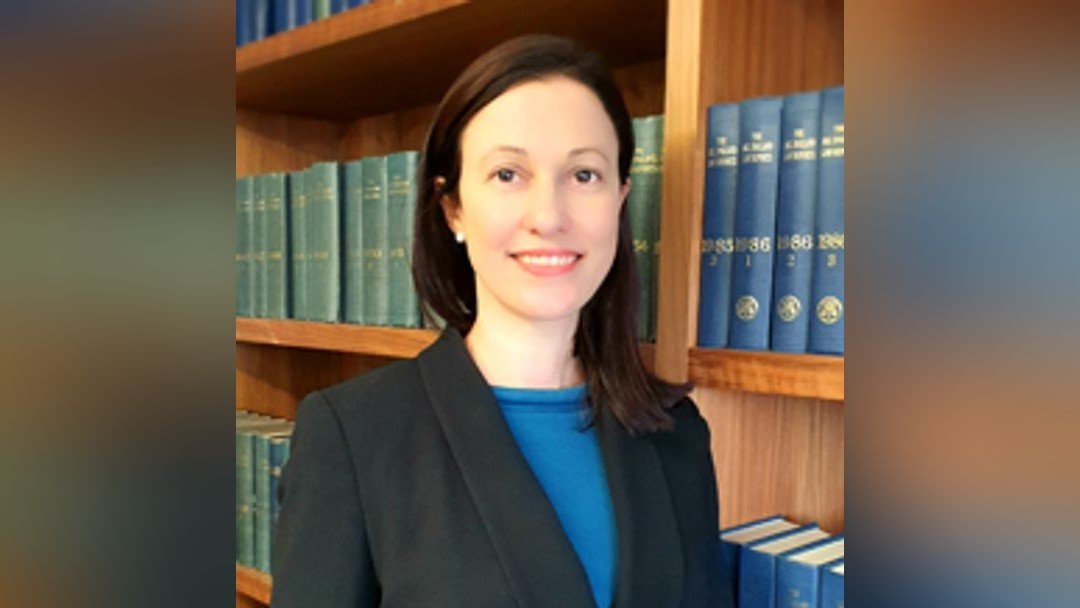
By Law News
A new study emphasises the need for clear guidelines in synthetic data creation to uphold transparency, accountability, and fairness, amid concerns about privacy and societal impacts
As synthetic data emerges as a promising alternative to traditional datasets, a recent study highlights the importance of establishing clear guidelines to govern its generation and processing. Synthetic data, created through machine learning algorithms from original real-world data, presents potential advantages in preserving privacy and overcoming limitations in data availability and quality.
Distinguished from real-world data, synthetic datasets are produced by algorithmic models like Generative Adversarial Networks or Bayesian networks. However, existing data protection laws, particularly those addressing personal data like the GDPR, pose challenges in regulating synthetic data processing comprehensively.
The GDPR's scope is limited to personal data, defined as information relating to an identified or identifiable natural person. While fully synthetic datasets are generally exempt from GDPR regulations, concerns arise when they contain personal information or pose risks of re-identification. This ambiguity regarding re-identification risk thresholds contributes to legal uncertainty and operational challenges in synthetic data processing.
Published in the journal Big Data and Society, a study led by Professor Ana Beduschi from the University of Exeter advocates for establishing robust accountability mechanisms and safeguards to ensure the ethical use of synthetic data. Key recommendations include implementing transparent procedures for accountability, preventing adverse societal impacts such as perpetuating biases, and promoting fairness in synthetic data usage.
Professor Beduschi emphasises the necessity of clearly labelling synthetic data and providing users with information regarding its generation process. This transparency is crucial, especially given the potential misuse of generative AI and advanced language models like DALL-E 3 and GPT-4, which can both train on and generate synthetic data, raising concerns about misinformation dissemination and societal harm.
By prioritising transparency, accountability, and fairness in synthetic data practices, the proposed guidelines aim to mitigate risks and foster responsible innovation. As synthetic data continues to evolve as a valuable resource, adherence to these principles can safeguard against unintended consequences and promote ethical advancements in data science.