Navigating the legal landscape of AI in manufacturing
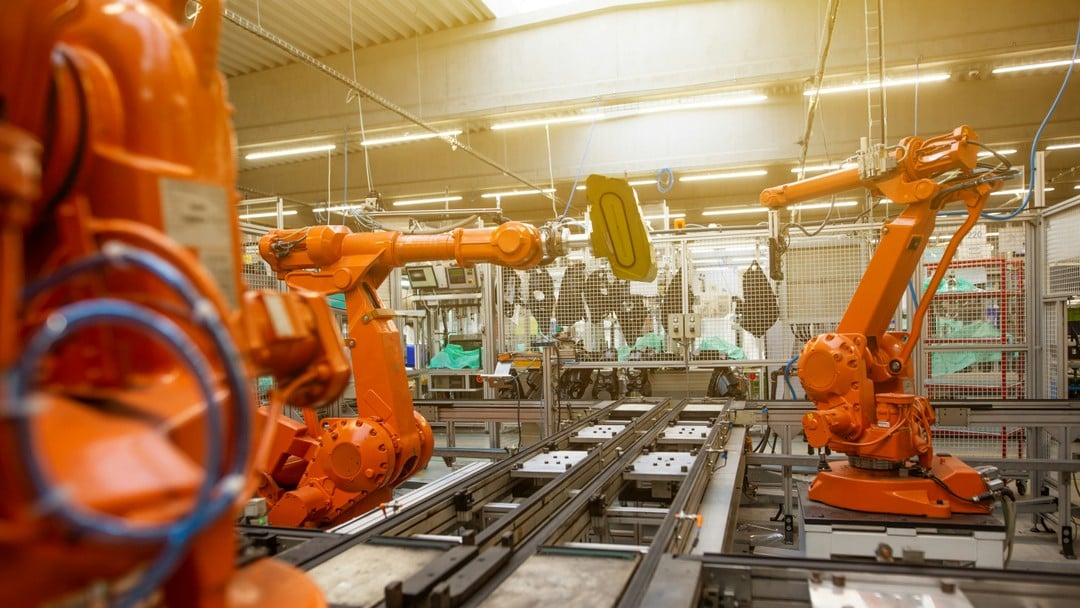
By Simon Key and Dominic Simon
AI is transforming manufacturing, but with innovation comes new legal risks, raising fresh considerations for practitioners
Artificial Intelligence (AI) is rapidly reshaping the manufacturing landscape, driving innovation across supply chains and revolutionising how products are designed, produced, and delivered. From predictive maintenance to automated quality control, AI is becoming a cornerstone of modern industrial operations. However, as these systems take on increasingly complex roles once managed by humans, the consequences of AI errors are potentially becoming more significant.
The UK legal framework currently lacks specific precedents addressing liability for AI-driven failures, leaving solicitors to interpret and apply existing legal principles to novel and technically complex scenarios. As AI adoption accelerates, legal practitioners must understand the potential risks posed to clients and be prepared to advise clients on how to mitigate legal risks.
The use of AI in manufacturing settings
AI is making a significant impact is the automotive industry, particularly at design and production stage. At the Toyota Research Institute, AI is being used to minimise design alterations by integrating engineering requirements earlier in the creative process. For example, designers can input text requests for specific design attributes, such as "sleek" or "SUV-like" based on a prototype and performance criteria.
AI is also thought to be enhancing quality control by removing or reducing the need for manual inspections, ensuring faster and more accurate product checks. Companies such as Tesla and BMW are using AI to inspect vehicle components before they leave the production line.
Another significant advancement is predictive maintenance, where algorithms monitor machinery in real time to help prevent breakdowns. General Electric has been using AI for this purpose in various industrial sectors.
Beyond production, AI is also being used to enhance supply chain monitoring. For example, Siemens' MindSphere is an Internet of Things platform that connects devices and machines across the supply chain. It is designed to enable real-time monitoring and advanced data analysis, enabling companies to track inventory, optimise logistics and predict potential disruptions, allowing for pre-emptive decision making and contingency planning.
When AI fails
While offering significant benefits in manufacturing, AI systems are not infallible and can make errors. For solicitors advising manufacturers, it is essential to understand these technical failure points, as they may form the basis of future disputes or claims.
Since AI outputs rely on data inputs, incomplete or biased data can lead to flawed outcomes. For example, an AI system trained on a limited range of product defects may miss new or uncommon issues.
Additionally, "overfitting" and "underfitting” can also lead to errors. Overfitting occurs when an AI model is too aligned with its training data, capturing irrelevant patterns that don't apply in real-world scenarios. Underfitting happens when the model is too simplistic, missing critical data signals.
Incorrect algorithmic assumptions can also lead to errors. For example, an AI-driven inspection system may assume that the surface texture of a metal part remains uniform throughout the production run. If a batch of parts has variations in texture due to a supplier issue or slight changes in production conditions, the system may fail to detect defects, leading to faulty products reaching the market. Similarly, AI systems designed to optimise efficiency may prioritise speed over quality, resulting in defects or product failures. This trade-off, if not properly managed, can lead to contractual breaches or product liability claims.
AI systems often interact with hardware, and faulty communication between the two can lead to issues. If an AI system becomes too advanced for the hardware on which it is installed, there may be unintended outcomes. Furthermore, human errors during AI training or deployment can also lead to mistakes. For example, applying an AI model built for one product type to a different type or failing to adjust AI programming to align with changes in quality standards could create issues.
Liability
The novelty of AI means that many jurisdictions have yet to establish clear laws on who is liable for AI-driven errors. As a result, the allocation of liability remains somewhat uncertain and highly fact-dependent. Liability may rest with the manufacturer that implemented the AI system, the software provider that developed it, or, in cases involving AI-driven robots, potentially the robot’s manufacturer if distinct from the software developer.
A key factor in determining liability will identifying the root cause of the error. If the fault lies in poor implementation or misuse of the system, liability may rest with the manufacturer. If the issue stems from the design or algorithm of the AI software itself, the software provider could be liable.
Establishing causation in these cases is likely to require expert technical analysis, and legal disputes may involve detailed forensic assessments of the AI system’s architecture, training data, and operational behaviour. Practitioners advising clients in such matters should be prepared to engage with technical experts early in the dispute resolution process.
In addition to the technical aspects of the failure, the level of control and oversight exercised by the manufacturer is likely to be considered. If a manufacturer heavily customised the AI system or operated it outside of its intended use, they might bear responsibility for the outcomes. On the other hand, if the manufacturer used an off-the-shelf solution from a reputable AI provider, the responsibility may shift toward the software provider, especially if the system’s failure was caused by a defect in the AI model or its training data.
The ability of AI systems to be continuously updated and modified, whether through human intervention or autonomous learning, adds further complexity. Manufacturers must ensure these systems are regularly monitored and maintained to minimise risk. However, accountability becomes more difficult to manage as systems evolve, particularly when updates are made by multiple parties or when the AI’s decision-making processes shift after deployment.
This evolving nature of AI raises important questions around foreseeability, duty of care, and the adequacy of monitoring protocols – all of which may influence how liability is determined. The current legal uncertainty could expose manufacturers to unforeseen risks, especially as precedents emerge. This is particularly problematic in a globalised manufacturing environment where AI systems are integrated across borders. Jurisdictions with differing legal standards may interpret AI-related liability in inconsistent ways, creating challenges for manufacturers who operate internationally.
AI liability is expected to be a key focus of regulatory bodies in the near future, with laws and standards likely to evolve as the technology matures. As such, practitioners need to anticipate and prepare for developments in AI-related legislation and work proactively with manufacturers to ensure that they comply with emerging requirements.
Mitigating risks
To mitigate the potential risks, practitioners should help manufacturers respond to potential liabilities before issues arise. Below are some non-exhaustive examples of potentially viable risk mitigation measures.
Contractual protections
Practitioners drafting or negotiating contracts with manufacturers should ensure that contracts with all relevant parties, including customers, clearly define the roles and responsibilities of all parties involved in the AI ecosystem.
These contracts should aim to limit the manufacturer’s liability wherever possible. Indemnity clauses can further safeguard manufacturers by shifting potential financial risks to other parties. However, the above clauses require agreement and are likely to be met with resistance, and even when agreed upon, may later be contested or interpreted differently, potentially leading to disputes, especially given the absence of established legal precedents governing AI-related liability. Practitioners should therefore draft these provisions with precision, anticipate potential areas of dispute and provide caveats to clients.
Reputable AI suppliers
Solicitors should recommend that manufacturers only work with suppliers with proven track records of delivering reliable, thoroughly tested AI solutions, to reduce the risk of errors. Solicitors can assist by conducting legal due diligence on suppliers and reviewing technical documentation to ensure that representations of system performance are clearly recorded and contractually enforceable.
Insurance
Traditional insurance policies may not cover AI-driven errors, so manufacturers should be advised to work closely with insurers to ensure that their policies address specific AI-related risks. Some insurers may be unwilling to cover such risks due to the evolving and unpredictable nature of AI technologies.
Gradual integration
Rather than immediately deploying AI systems across the entire manufacturing process, it may be useful for manufacturers to consider a phased approach to integration such as pilot projects or limited deployments. This can help to monitor its performance in controlled environments before real-world conditions, allowing manufacturers to build confidence in the AI system's reliability and identify potential issues early on. Solicitors can support this approach by structuring phased implementation clauses in supplier agreements.
Stress testing
Manufacturers should conduct thorough stress tests and scenario simulations to evaluate how the system behaves under different conditions, to identify potential vulnerabilities in the AI system that may not otherwise be evident during everyday use.
Ongoing monitoring and improvement
Given that many AI systems are dynamic—capable of learning and adapting over time, it may be prudent to advise manufacturing clients to conduct or commission regular audits to ensure that AI systems continue to operate as intended.
Solicitors can also assess whether these audits reveal any potential issues in complying with contractual obligations and regulatory standards, or weaknesses that may lead to AI errors. Input from technical experts may be necessary.
Manufacturers can also work closely with AI providers to routinely update, calibrate, and refine the software. Solicitors can play a key role in ensuring that key responsibilities are clearly defined in contracts and that any changes to the system are properly documented and risk assessed.
Education and training
Workers interacting with AI systems should receive appropriate training and education to understand how the technology works, its capabilities, limitations and the potential risks associated with their use, to prevent misuse and overreliance on AI, particularly in situations where human oversight and judgement are critical. In some cases, further or regular training may be necessary.
Employment law practitioners can support manufacturers by developing clear acceptable use policies and implementing robust disciplinary procedures to address inappropriate or negligent use of AI systems. These measures help reinforce accountability and ensure that AI is deployed responsibly within the workforce.
Final thoughts
The integration of AI into manufacturing presents significant opportunities for innovation, efficiency, and quality improvement. However, it also introduces complex legal and operational risks, particularly as AI systems become more autonomous and embedded in production processes. For practitioners, this demands a proactive and multidisciplinary approach where possible.
As legal frameworks continue to develop, practitioners should stay informed about emerging legislation, regulatory guidance and case law. By doing so, they can help clients not only mitigate risk but also harness the full potential of AI in a legally sound and commercially strategic manner.