Don't believe the AI hype
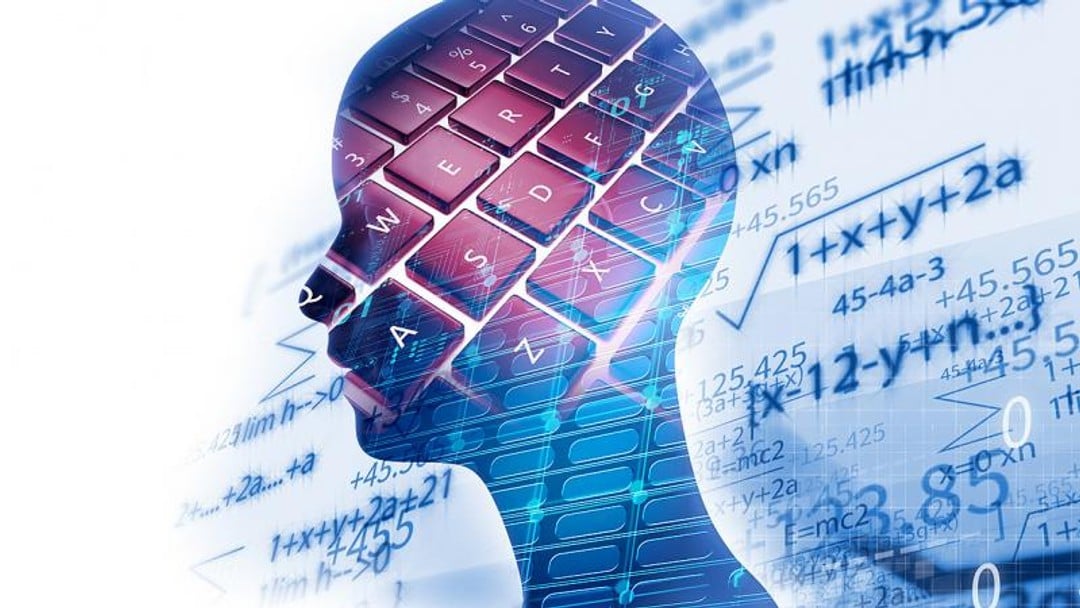
Martyn Wells separates the facts from the science fiction when it comes to the role of artificial technology in the legal sector
You can barely read a legal technology article these days without some reference to artificial intelligence or machine learning. The terms have almost become interchangeable and there are some fantastic articles discussing how these technologies are going to disrupt the legal industry.
So let’s separate the science facts from the science fiction.
Artificial intelligence
I’ve asked three professors of artificial intelligence from leading UK universities for their definition of AI. So far the best answer is: ‘Every year we decide what it should be.’ It doesn’t really help.
In 1943, Warren McCulloch and Walter Pitts produced a paper, ‘A Logical Calculus of the Ideas Immanent in Nervous Activity’, which demonstrated how a Turing machine could produce an electronic model of a brain. This is widely accepted to be the first work in the AI field.
It wasn’t until the late 1990s that computational power became affordable and the first practical AI developments took off. IBM developed a supercomputer to play chess, Deep Blue. Its computational power had access to 700,000 previous grandmaster chess matches and used brute force to compute the best move. Garry Kasparov was defeated and a whole generation was inspired by the power of computing.
Today, AI applications have barely changed: create your ontology from business logic, add your big data set, and you will create an outcome based on the hard limits of your ontology. Static program instructions will only ever produce predictable outcomes.
Machine learning
ML is another iteration of AI that split away from the mainstream in the 1980s. It gives an application the ability to ‘learn’ without being explicitly programmed to do so. Unlike AI, decision making is not necessarily static, and a machine-learning application learns from its past predictions. The more predictions ML makes, the more accurate its results will become; its algorithm becomes self-healing.
ML captured the public imagination in March 2016, when Lee Sedol, a renowned Go champion, was defeated by AlphaGo, Google’s purpose-built machine-learning program.
Common applications use ML as a method to help identify data that may contain variables around a common entity. If you think about intrusion detection systems seeking to defend against cybercrime attack vectors, or a speech recognition system translating dialect as well as language, you will start to see how these applications learn from their actions.
Machine learning has its roots in data-mining techniques common to business intelligence applications used to analyse big data. This becomes relevant to the legal sector when applied to knowledge management, e-discovery, or contract reviews, and there are already some great tools available to do this. ML applications organise your data into clusters, review the outcomes against objectives, and learn from the accuracy of its decisions.
Deep learning
DL also has its roots in AI, with the first working algorithm appearing in 1965 and theorised by McCulloch and Pitts in their 1943 work. DL is vastly complex; applications are constructed to model high-level abstractions from within a dataset.
The ability to offload complex calculations to graphics processing units and the availability of large stores of unstructured data have driven the demand for less structured analysis and DL. Complex mathematical algorithms are constructed to manage multiple inputs and outputs that are distributed across a model. These factors are then organised into layers in a hierarchical fashion and data then transformed across these layers. New methods of analysis are emerging all the time; pretty soon DL will get the attention it deserves.
DL neural networks are the best method of identifying patterns in unstructured data. Take the pixels produced on your mobile phone camera, and how Snapchat can model your face and apply a silly filter. It’s all DL. What about the tech being built into autonomous cars to recognise other vehicles, inanimate objects, and pedestrians, or even read speed limit signs? DL too.
By now you’ll see AI, ML, and DL are not new. What we do have that is new is the computing power and data to make best use of these techniques.
The legal world will not yet be fronted by chatbots and virtual reality robots dispensing legal advice: we have too much of a cultural legacy on passing the Turing test. Instead these tools are going to break the back of research, knowledge management, and complex drafting. They are going to predict your propensity to succeed, your likely fee from a matter, and advise on risk exposure, as well as prompting activities in the new world of case management.
So, focus on your outcomes and identify where AI will help your firm. Be very clear of these before you get seduced by the heady intellectualism of the products themselves.
Martyn Wells is IT director at Wright Hassall
@Wrighthassall www.wrighthassall.co.uk